Event detection or identifying disruptions in the city’s economic, social or behavioral patterns are of great interest in Urban Informatics. Social media data offers the potential to understand trends and patterns, real-time monitoring of disruptions and events, as well as discovering sentiments, perceptions and beliefs. Yet, almost all social media data, whether it is microblogging data, or website data, are noisy and a mixture of text and images, and with provider restrictions and sampling restrictions to access.
Several recent and ongoing streams of work involved information retrieval and analytics of social media data sources including Twitter, WeChat, and website data. In “The Geography of Human Activity and Land Use: A Big Data Approach” WeChat data is used to understand the activity and land-use patterns in Beijing, China. In “Sensing Spatiotemporal Patterns in Urban Areas: Analytics and Visualizations using the Integrated Multimedia City Data Platform”, Twitter data is used to understand urban metabolism in Glasgow, UK. In “Beyond Geo-Tagged Tweets: Exploring the Geo-Localization of Tweets for Transportation Applications” and “On Fine-Grained Geo-Localization of Tweets”, a location prediction approach is performed to geolocalize non-geotagged tweets to increase the sample size of tweets, and to identify places with social hazards in the City of Chicago. In “Digital Infomediaries and Civic Hacking in Emerging Urban Data Initiatives”, by analyzing company websites, we identify new forms of businesses that are increasingly providing urban data-driven services in cities, thereby disrupting urban economies.
Although the data are noisy, using web and social media data provides an organic and natural measure of events and patterns in the city. The possibilities of such insights are especially higher if we have more geolocalized data, i.e., not just an understanding of the contents of a Tweet, but where the user was when they tweeted, or where the object of a tweet is located. However, the reality is that such geolocalized data are sparse for the type of robust data needed for event detection (e.g. “there is an traffic crash in XYZ location”, or “there is a street protest in a certain location”). One strand of work we have focused on is to predict the locations of non-geotagged Tweets, thereby increasing the sample sizes of tweets available for location-specific event detection.
For example, in our analysis of Chicago-area geotagged versus geolocalized (using our own algorithm) tweets, we find that using geolocalised tweets allows discovery of a larger number of incidents and socioeconomic patterns that are not evident from using geotagged data alone, including activity throughout the metropolitan area, as well as those in deprived “Environmental Justice” (EJ) areas where the degree of social media activity detected is usually low. This can be seen from Figure 1. The figure on the left shows a map of the Chicago-area region using user-geotagged Tweets only. The map on the right is of the same region but with user-geotagged Tweets as well Tweets which are not geo-tagged, but which we estimated as being located in the same area using our geolocal prediction algorithm.
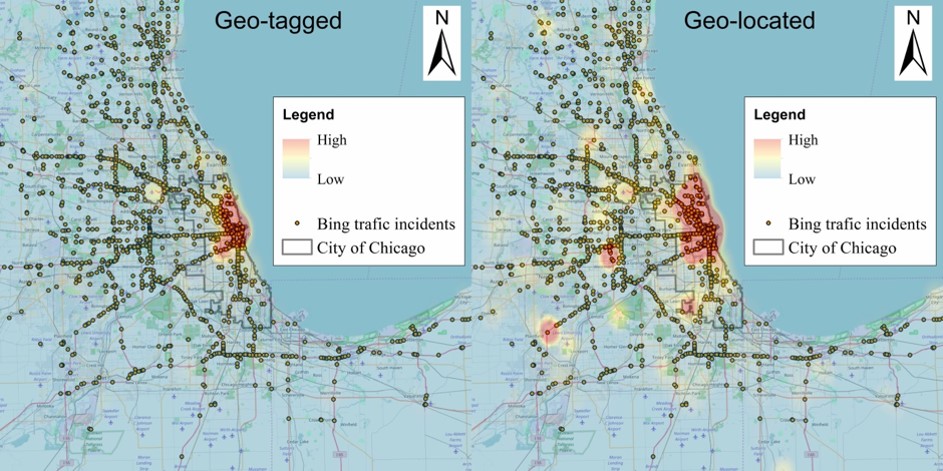
Figure 1: Geotagged vs geolocated Tweets in the Chicago metro area
Using social media data therefore has the potential to open up and offer residents avenues to data justice which is not possible from traditional sources such as administrative records, law enforcement data, healthcare data, and surveys. However, one needs to exercised caution as well, due to the many other concerns associated with social media and web data previously identified in the literature.
References
- Wu, W., W. Liu, P. Thakuriah and J. Wang (2020). The Geography of Human Activity and Land Use: A Big Data Approach. In Cities: The International Journal of Urban Policy and Planning. Vol. 97, February. Early online: https://doi.org/10.1016/j.cities.2019.102523
- Paule, J. D. G., Y. Sun and P. Thakuriah (2018). Beyond Geo-Tagged Tweets: Exploring the Geo-Localization of Tweets for Transportation Applications. In Transportation Analytics in the Era of Big Data, pp. 1-21. DOI https://doi.org/10.1007/978-3-319-75862-6_1
- Paule, J. D. G., Y. Moshfeghi, J. Jose and P. Thakuriah (2017). On Fine-Grained Geo-Localization of Tweets. Proc ACM SIGIR conference, Amsterdam, Netherlands, (ICTIR’17), pp. 313—316, ACM. doi https://doi.org/10.1145/3121050.3121104
- Thakuriah, P., L. Dirks, and Y. Keita Mallon (2017). Digital Infomediaries and Civic Hacking in Emerging Urban Data Initiatives. In Seeing Cities through Big Data: Research, Methods and Applications in Urban Informatics, pp. 189-208, Springer, NY. doi https://doi.org/10.1007/978-3-319-40902-3_11
- Thakuriah, P., K. S. Nowicka and J. D. G. Paule (2016). Sensing Spatiotemporal Patterns in Urban Areas: Analytics and Visualizations using the Integrated Multimedia City Data Platform. In Journal of Built Environment, Vol. 42(3), pp. 415-429. doi https://doi.org/10.2148/benv.42.3.415